Hybrid Deep Learning Model for Traffic Flow and Speed Forecasting at Urban Junction
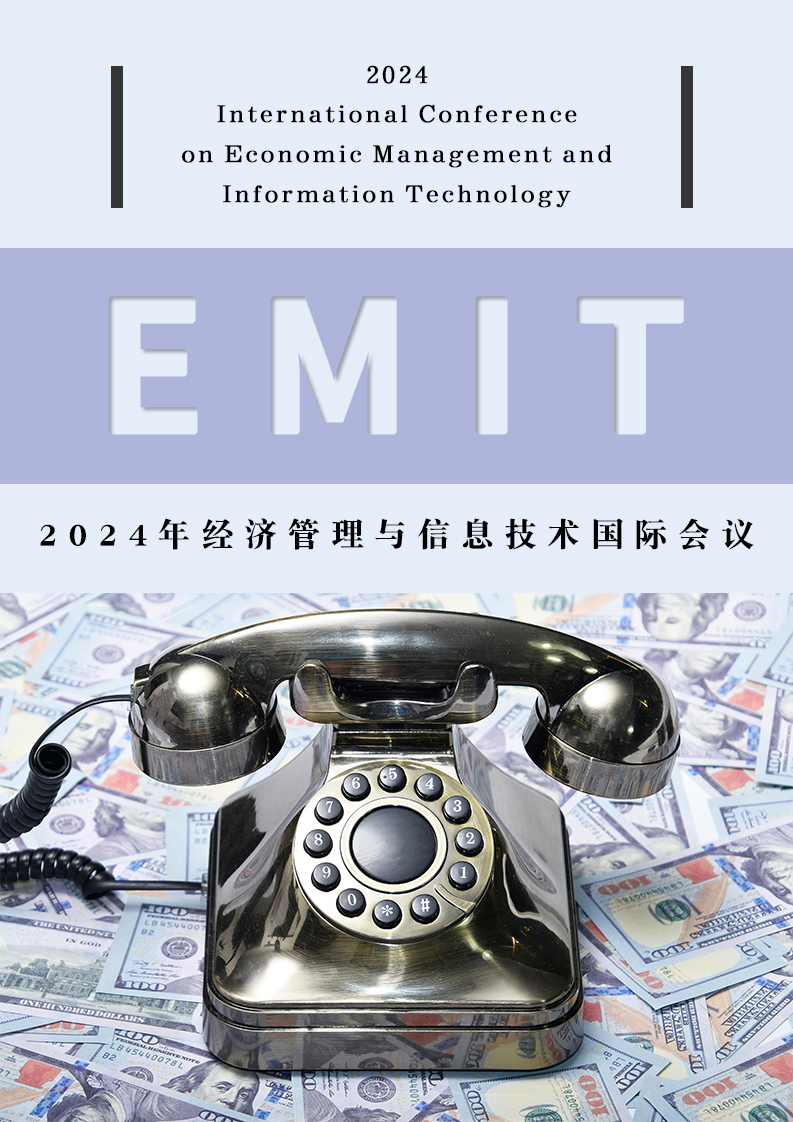
Authors:
Li Ma, Yongshun Wang, Xiaoshi Lv, Yongheng He, Guixin Wang
Keywords:
Intelligent Transportation; smart city; traffic Prediction; deep Learning
Doi:
https://doi.org/10.70114/aimedr.2024.1.1.P48
Abstract
The hybrid deep learning model H-CNN-LSTM (Hybrid Convolution Neural Network and Long Short-Term Memory) is introduced in this paper. The model combines CNN to extract traffic flow and speed features, LSTM networks to model temporal dependencies, and employs a combined matrix and a rolling forward input method during data input, enhancing the coarse-grained extraction of traffic data and further refining the utilization of adjacent data. Our research focuses on the complex dynamic traffic conditions of Fuli East Road in Xigu District, Lanzhou City of China. Using detailed traffic video data collected during one month of evening rush hours, we predict future traffic using the H-CNN-LSTM model. By comparing it with other prediction methods, we validate the effectiveness of our approach in predicting traffic flow and speed in real-world traffic scenarios