Contrastive Knowledge Graph Error Detection Model of TransR Based on Spatial Transformation
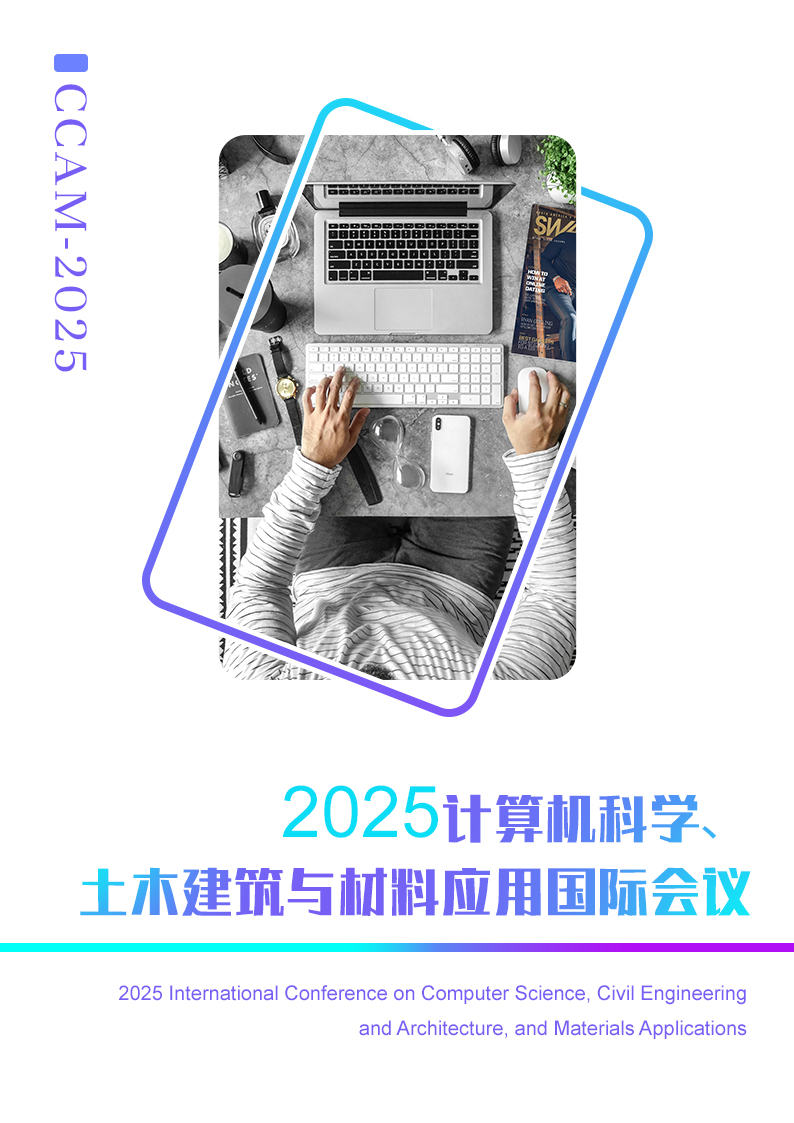
Authors:
Jiawei Fan, Yifeng Yue, Xianghui Ren
Keywords:
Knowledge Graph, Error Detection, Space Transformation, Contrastive Learning
Doi:
https://doi.org/10.70114/acmsr.2025.2.1.P370
Abstract
Contrastive Knowledge Graph Error Detection (CAGED) is designed based on the contrastive learning of knowledge graphs. CAGED employs an error-aware knowledge graph neural network (EaGNN) that utilizes a gated attention mechanism to inhibit the spread of information from incorrect triplets. However, EaGNN's architecture does not account for the transformation of entities and relations across different spaces, thereby limiting the expressive capacity of the model. Furthermore, CAGED uses a static balance parameter to modulate the impact of contrastive loss and embedding loss, which relies heavily on human expertise and increases the difficulty of model training. The paper introduces contrastive knowledge graph error detection model of TransR (TransR-CAGED), an enhanced EaGNN approach that maps entities into relation-specific spaces, thereby capturing intricate interactions among triplets. It features a dynamic balance parameter informed by the variances in triplet representations across different perspectives, enabling the dynamic adjustment of weights assigned to contrastive loss and embedding loss. This innovation renders model training more reliable. Compared to the conventional CAGED method, the proposed strategy exhibits distinct advantages, especially in scenarios where high recall rates are critical