Data Mechanism-Driven Modelling of Environmental Thermal Errors in Large Gantry Five-Axis Machine Tools
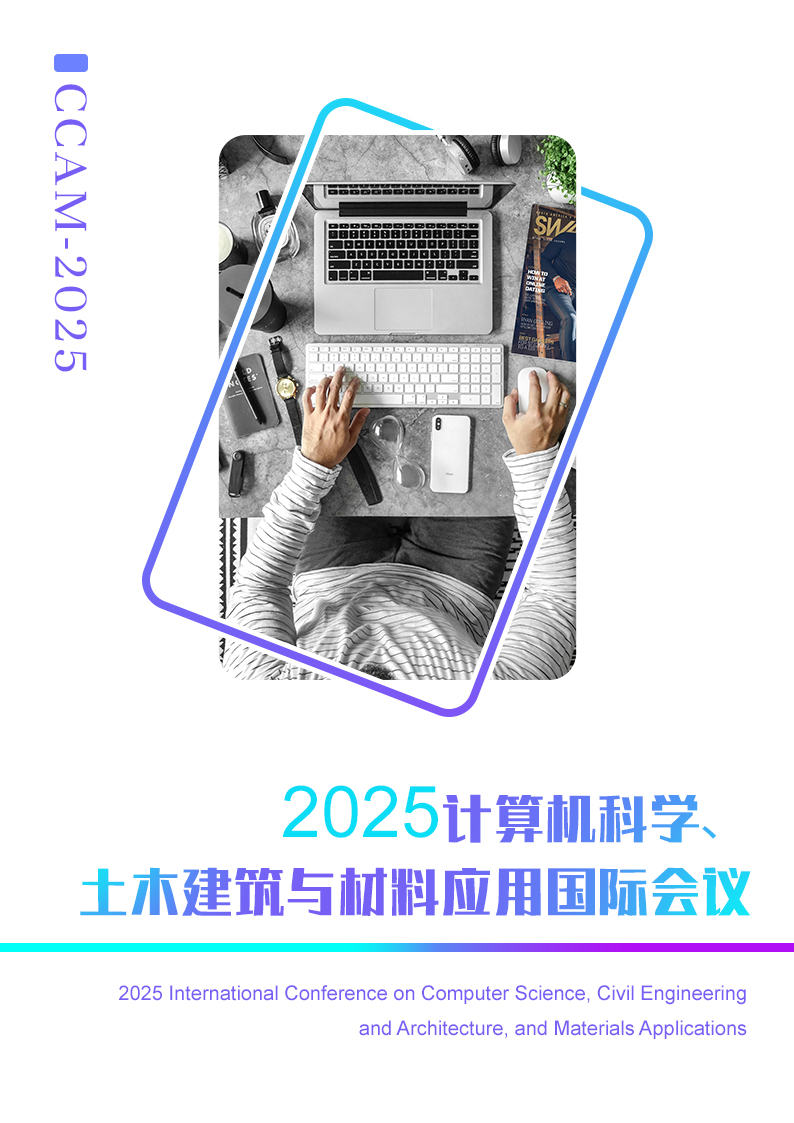
Authors:
Shi He, Zhi Huang, Jianhong Sun, Jianjun Tang, Mingxin Qiao, Haonan Xiong, Xianglong Li
Keywords:
Large gantry machine tools; Ambient temperature; Thermal error modelling; Data- and mechanism-driven; Short- and long-term time neural networks
Doi:
https://doi.org/10.70114/acmsr.2025.2.1.P364
Abstract
Thermal deformation in large gantry five-axis machine tools significantly impacts machining precision. This study examines the influence of environmental temperature variations on thermal deformation in such machines. To improve the accuracy of predicting integrated environmental thermal errors, a Grey-Enhanced Long Short-Term Memory network with Physical Mechanism Modeling (GELPM) is introduced. The research focuses on a large gantry machine tool, combining finite element simulations with experimental analysis to study thermal drift errors at the tool tip caused by ambient temperature fluctuations. A comparative analysis of GELPM, CNN-LSTM, and GNNMCI(1, N) models is conducted. The results reveal that GELPM outperforms previous neural network models by addressing challenges of limited data and time-series prediction, offering enhanced prediction accuracy and robustness